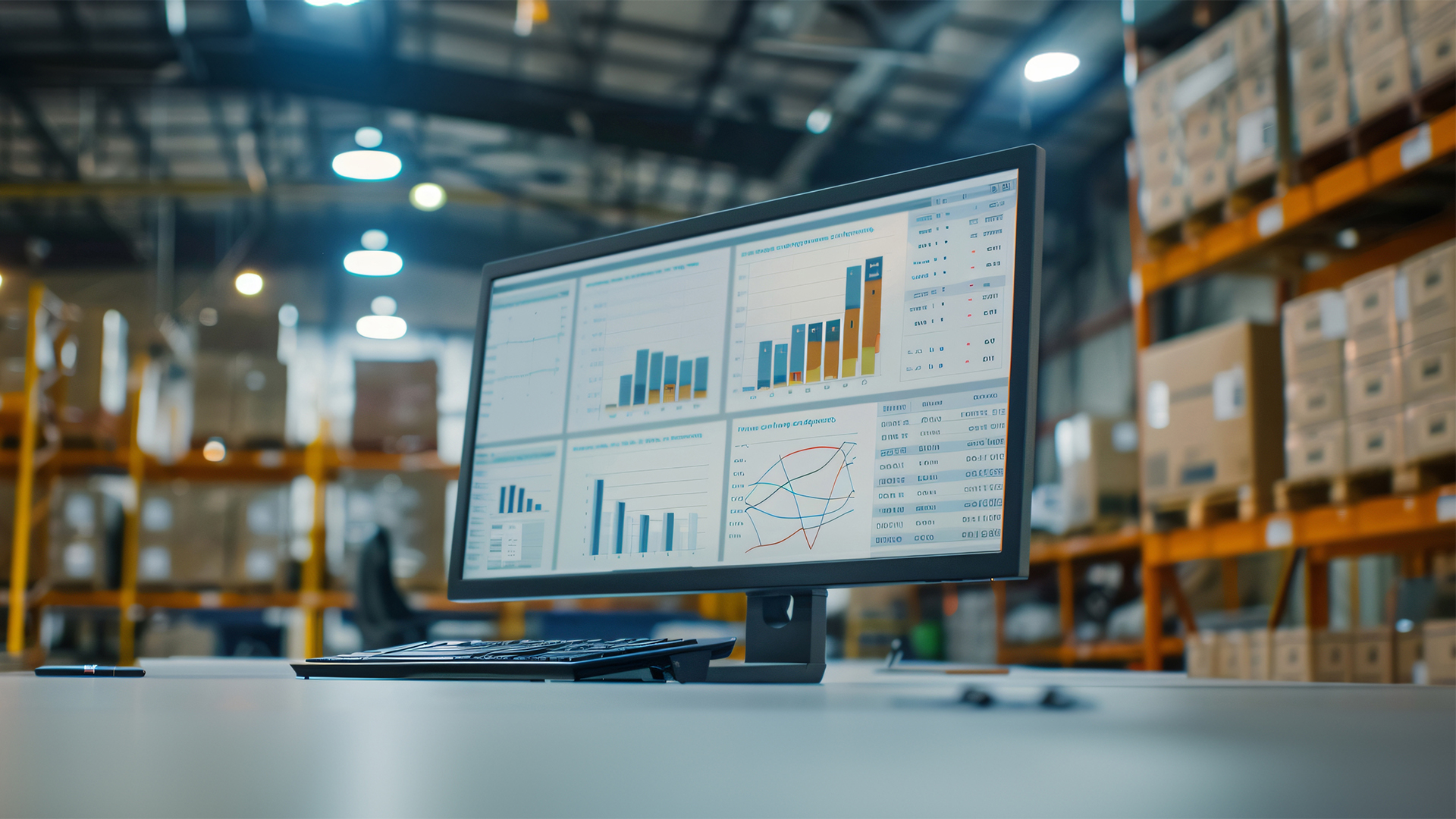
Data-driven insights can help optimise the performance, maintenance and sustainability of warehouse automation. Dan Migliozzi, Sales & Marketing Director, at independent systems integrator, Invar Group, sets out how to achieve the best results.
Current materials handling and intralogistics equipment is amazingly reliable. Nonetheless, there is a lot to go wrong – all those mechanical parts like rollers, bearings, motors, belts, not to mention switches, sensors and the rest of the electronics. For many businesses this equipment is fundamental – if it’s offline, everything stops.
Unexpected failures, and unplanned maintenance and repair, don’t just increase costs and impair customer service, they have direct and significant environmental and sustainability impacts. But by implementing data driven maintenance strategies these cost, performance, and environmental impacts can be greatly reduced.
Don’t be blinkered
Some companies, particularly those with limited in-house capabilities, work on an ‘if it ain’t broke, don’t fix it’ basis. This may appear to reduce unnecessary downtime and cost, but is a high-risk strategy. There’s a well-known law that states if something can fail, it will, and at the worst possible moment – peak season, rush order, Bank Holiday weekend when the spare parts stockist is closed. Not recommended.
A more sophisticated approach is that of planned, scheduled maintenance. Components subject to wear, or otherwise likely to fail, are replaced at regular intervals – as recommended by the equipment manufacturer, or based on bitter experience. This approach too has disadvantages.
The expected life of a part is a statistical construct – some will fail early; others may be good for much longer. Maintenance intervals are often based on the calendar, rather than the amount and nature of the usage the equipment has experienced – typically, all the parts of a given ‘lifespan’ will be replaced whether they need it or not. Perfectly good parts are sent for scrap. Meanwhile, the performance of other components may be degrading, well in advance of their ‘due’ replacement date. This may have knock-on effects on the condition or life of other system components, while increasing the consumption of energy, lubricants and other consumables. None of this is good for sustainability.
An intelligent data-driven approach
Maintenance doesn’t have to be this arbitrary. Most materials handling automation gathers a plethora of condition monitoring and other data that can be used in a preventative maintenance approach – key parameters, perhaps the energy consumption of motors, or the temperature of bearings, can be monitored, and generate alerts and warnings before the worst happens.
But instead of maintenance staff merely reacting to warnings that an element is, or is about to go, out of its performance envelope, we can use intelligent analytical software to drive the maintenance process in the most efficient and sustainable directions.
We can bring together both historical and real-time data, from SCADA and other systems, to identify failure areas and causes – both one-time events and regular wear-and-tear, mean times between failures, and downtimes required to take action. We can use data on actual loadings and usage, rather than elapsed times, to predict which components are likely to require replacement and when – and which identical components should still be okay. All the sites we instal have this data waiting to be used and we have the software tools capable of analysing this data, to inform our decisions on the most appropriate, proportionate actions to take.
Further, software empowers learning, encouraging continuous improvement and potentially revealing where investment in new equipment, or appropriate upgrades and enhancements – or indeed staff and operator training – may be needed.
Data driven maintenance mean that equipment can operate longer at maximum capacity, and reduce those minor jams and other incidents, while necessary downtime can be optimised to suit patterns of work. This makes best use of engineering staff (internal or external), to anticipate the need for, and ensure the availability of the necessary spare and replacement parts so that maintenance downtime is not wasted.
Sustainability strategies
Data analysis of warehouse automation and its maintenance needs contributes to a wider suite of environmental goals and strategies.
Analytics allows for efficient use of a most critical resource – planning where and when trained staff will be needed, and what their training needs are.
Effective maintenance strategies support waste reduction goals by reducing the unnecessary use of costly (in economic and environmental terms) replacement parts. Parts may be recovered when they are still able to be reconditioned rather than scrapped.
Data driven preventative maintenance ensures efficient performance of the automation, thus reducing consumption of energy and consumables – a badly worn conveyor belt may consume 2-6 times as much energy as one in good condition. More generally, analytics can be used to drive the automation in the most energy-efficient modes.
The consumption and waste of packaging materials and their contents, damaged by underperforming or failed equipment, is reduced. Automation also reduces or eliminates the use of more polluting forms of materials handling equipment such as lift trucks.
Automation can mitigate or eliminate many of the Health & Safety risks associated with warehouse operations, such as lifting. Equipment that is well maintained so as to stay within its designed operational envelope is inherently safer.
Importantly, analytics can reveal differences in the lifecycle impacts of parts and materials from different suppliers, which can help inform sustainable procurement policies.
And whilst the physical maintenance operations inevitably incur downtime and another round in the age-old battle between operations and engineering, machine monitoring means the need to stop the line for inspection and assessment is largely eliminated. Ironically, disassembly of equipment for inspection is itself a recognised cause of failure!
We are all rightly concerned about the sustainability of our companies’ operations. Intelligent warehouse automation supported by a data analytical approach to maintenance which predicts and prevents equipment failures, will reduce downtime, improve costs and service levels, and significantly reduce the environmental impact of operations, maintenance, and repairs.
Further independent advice on transforming operational performance in the warehouse can be found at: www.Invargroup.com