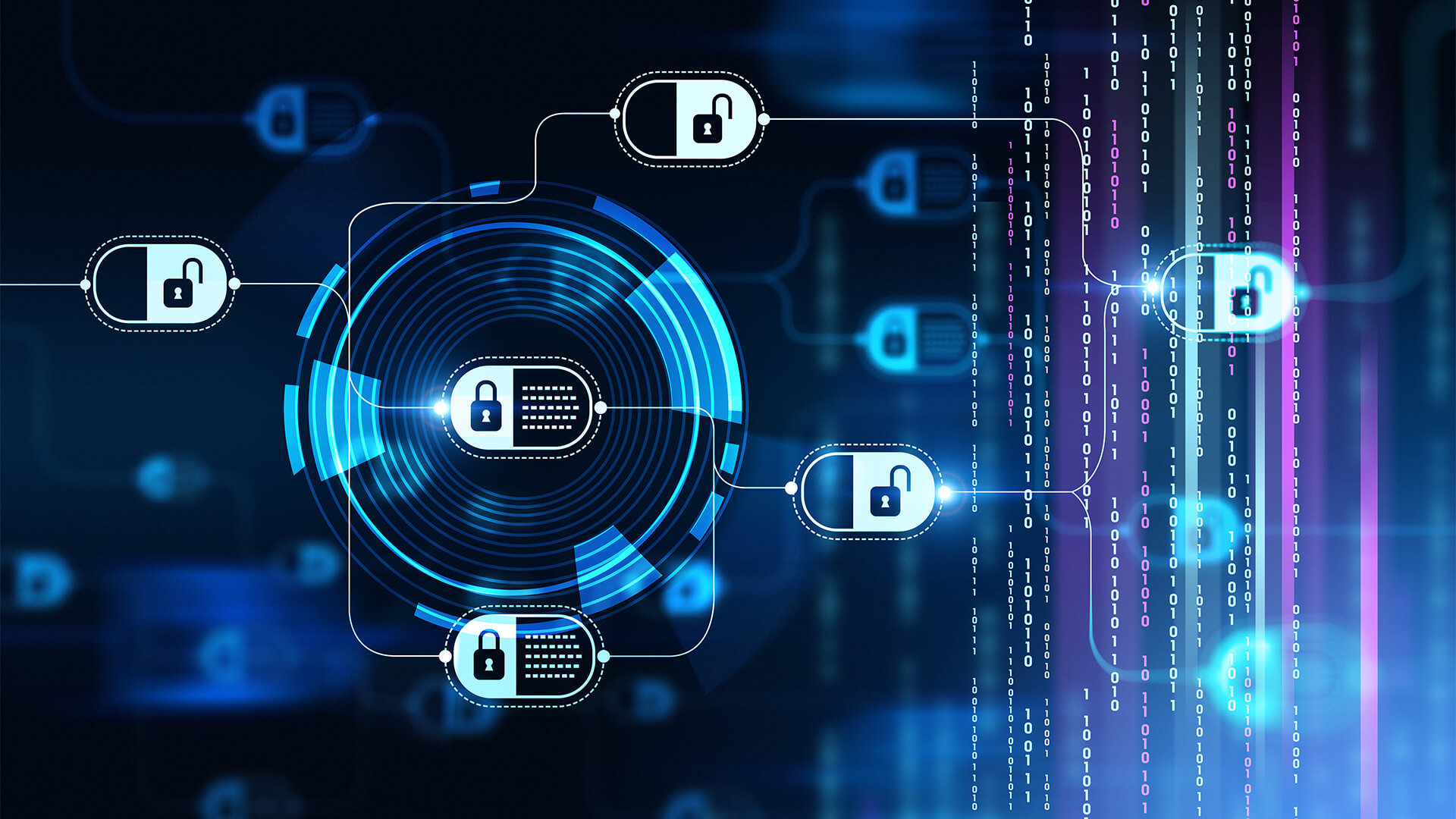
Artificial intelligence (AI) has emerged as a significant player in today’s business models. More organizations are looking for ways to reduce human intervention in their operations as much as possible. This results in a smoother, uninterrupted, 24/7 system that operates like human intelligence, but is free from fatigue and errors common with human resources.
However, building an effective AI ecosystem isn’t a one-off thing. Like human intelligence, your AI systems learn and become better with time. This means that you have to make the right strategy and have enough data from which your machines can learn. Over time, the system becomes better and will start performing as expected.
This article will look at how you can build an effective AI ecosystem that works and delivers the desired results. Read on for the details.
1. Outsource to Experts
Developing your own AI ecosystem can be quite a challenge, especially if you’re not knowledgeable on the subject or have the right resources to get all the systems in one place. Because an AI ecosystem is a huge investment, you need to make sure that it’s running at full capacity for it to pay off.
One way to do this is to outsource the development of the AI ecosystem to experts and professionals. Several companies, such as cnvrg.io, among others, can help you develop, train, manage data, and deploy your AI systems in any infrastructure. It’s advisable not to build an AI ecosystem from scratch yourself because it’s unlikely that you’ll have the resources and capabilities required for this undertaking. If this isn’t done correctly, you’ll waste time, money, and effort.
2. Standardize Features
It’s important to make features standardized and repeatable to build an effective AI ecosystem. The term ‘feature’ refers to a single function or aspect of a program designed for a specific purpose. For example, one of the features of a smartphone might be its ability to send text messages. If the feature is standardized, it’ll work in the same way no matter what device it’s applied to.
To make features standardized, they need to be used by multiple teams working on different projects. A feature should be repeatable so that different developers can use it without having to modify any of the code behind it. This means that they can focus on their projects and not worry about how these features are implemented in other areas.
Moreover, standardizing your features will help you identify gaps or duplicated efforts as the ecosystem grows. This will ensure that you can adjust accordingly and keep your AI ecosystem at an optimum point.
3. Manage Data and Data Security
Your biggest challenge with AI is creating and maintaining the right data ecosystem. Data is the key part of AI because machines learn and improve based on the data you feed them. So, the data should be correct and secure from any corruption. If you don’t have good data, no algorithm can help you.
The most common problem organizations face with AI is not having enough of the right kind of data to train their algorithms. Inaccurate or incomplete data can negatively affect how an algorithm performs. If it isn’t trained on enough examples for each type of prediction it needs to make, it may be less accurate than if it had been trained on more examples or a wider variety of examples.
Ensure you know where your data is coming from, who has access to it, and what security measures are in place. For example, suppose you collect sensitive user information, such as credit card numbers or Social Security numbers. In that case, you need to ensure that you have adequate security measures in place so that this information doesn’t get compromised.
4. Promote Responsibility Through Risk Assessment
The lack of transparency around algorithmic decision-making could be a liability. Algorithms are only as ethical as the input data you feed them and the goals you task them with. The algorithms may be complex, but the decisions they make don’t have to be.
Thus, for your organization to build and implement AI safely, you should have a documented risk assessment process. By creating a culture of transparency, accountability, and mutual respect, you can build an effective AI ecosystem within your organization that won’t derail your business, or harm your customers or employees. You’ll also put yourself in a position to thrive in this new era of technology that requires new ways of thinking.
Conclusion
Getting different AI systems to fit into one ecosystem and perform to achieve one common goal can be challenging. It requires training, data management, deployment, and trials before getting it right. However, letting experts build it by documenting your risk assessment, among other practices discussed in this article, will help you get it right.