While ChatGPT become lazy recently, denying to perform basic tasks, and making excuses on why it shouldn’t do, what was required, it is still hard to deny that machine learning models can bring many advantages to any technological solution, and FinTech is not an exception.
To understand what should we expect from different modern natural language processing models, and how they can change financial technology for good, we have to learn its basics first.
Machine Learning Algorithms in a Nutshell
Such digital technology empowers and enables data-driven decisions by training with the use of vast amounts of data for training.
Any AI can be trained to serve a certain purpose or switch the field of business processes by changing the data basis. Potentially, your virtual assistant can become an industry-agnostic instrument, if its advanced algorithms get access to a wide range of data from different spheres.

The newest technology still has limitations and can be used in just a few industries. Modern hardware capabilities do not support the full power of AI-driven technologies, and nobody knows when we will use the full potential of such automation tools. Still, this is mainly related to industry-agnostic AI solutions.
On the other hand, modern niche-specific AI assistants can reach great results, when it comes to distinct industries like the FinTech sector. For a better illustration, let’s examine the capacities of AI for Financial institutions.
Artificial Intelligence in Financial Products
Thanks to such innovative solutions, specialists from the financial sector got a chance to improve several different KPI metrics results and automate countless services and processes.
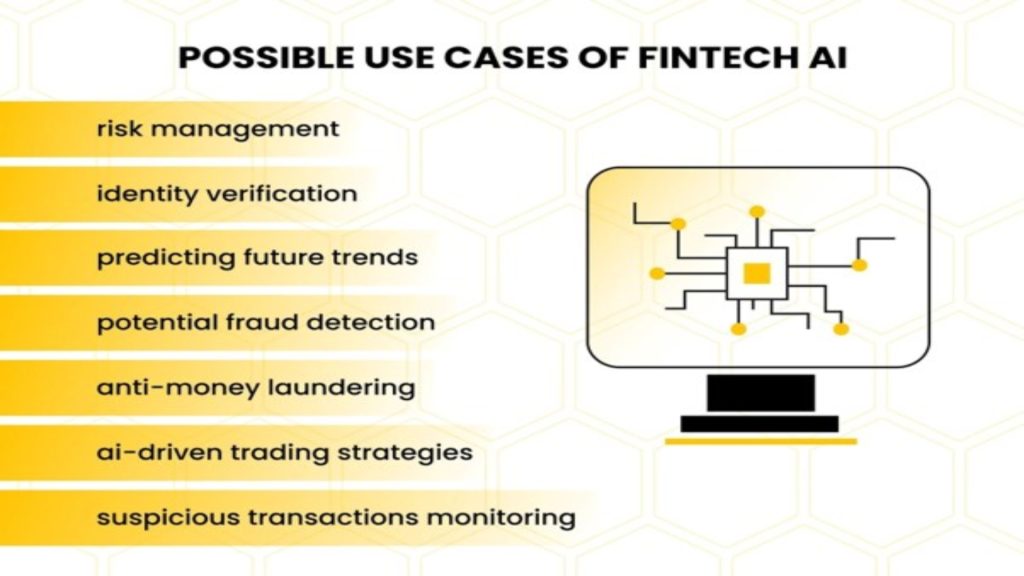
It includes such complex and complicated tasks as risk management, potential fraud detection, suspicious transactions monitoring, or identity verification, and even proposes such advanced services as creating AI-driven trading strategies, based on actionable insights and predicting future trends, or anti-money laundering. All the foregoing helps banking institutions to save billions of dollars and earn customer trust by bringing next-era security measures for fraud prevention.
AI is mainly data-driven. However, it proposes a few other essential features, related to working with vast amounts of information – predictive analytics and pattern recognition. Therefore, it can be used to automate all data-related processes.
Pattern detection with machine learning plays a pivotal role in all previously mentioned features. It can also be used for making financial decisions, based on valuable insights and recent market trends, which can help with further predictions like sales forecasting, which can bring a lot of value to your investment strategies.
AI-Powered Fraud Detection for Finance Industry
Fintech companies have to constantly deal with different financial crimes, starting with identity theft, and ending with false positives, etc.
The most powerful feature of artificial intelligence is the automation of data management and analysis when it comes to monitoring fraudulent activities.
AI solutions are capable of distinguishing templates. For instance, it can build predictive models of user behavior, which helps not only to boost personalized experience by providing them with the most suited bonuses or other proposals but can help with defying bad actors as well.
To enable such a feature, you will have to spend a lot of time and resources, i.e. such advanced technologies still require training and data access.
How to Train AI-Powered Fraud Detection Bots?
If you are willing to track fraudulent transactions efficiently, it is not enough to simply integrate an AI-powered assistant.
You can use open-source solutions like ChatGPT for financial transaction analysis. However, it might be less efficient, than you would expect.
The core issue with such ready-made solutions is the fact, that mostly you won’t have access or direct possibility to impact and change its learning curve by adjusting the data, it is based on.
It is essential to understand how to train your artificial intelligence for the banking industry. Fortunately, there is one vital aspect, which is common for any such industry-specific training.
Data Access
Any Machine learning starts with choosing the data sources, which will be used for AI training.
Before the bot will be able to distinguish patterns, you should teach him what it is, and show some examples of what to look for. In other words, you will need to give it access to a database, which includes very precise models and data units.
Transformation From General to Niche-Specific AI
You can extend the accessed data, increasing the efficiency of such training. After you have trained your AI to define patterns, you have to deepen its knowledge and transform it into a niche-specific.
By giving AI access to credit and transaction history, financial institutions can easily achieve two goals at once: proceed with their digital transformation and enable AI-powered financial analytics without human intervention.
This part is very tricky due to several factors:
- such integrations can impact the overall security of the whole financial market
- it is unclear how legal such software upgrade is: each country, as well as different international organizations, have their regulations and requirements.
The best way to deal with both issues – is to communicate with government agencies and lawyers to learn and calculate all the risks and laws.
What Technologies to Use for Development?
Python programming language seems to be an ideal option for such tasks. It is one of the most popular solutions for artificial intelligence, machine learning, and FinTech app development. Python is very easy to learn, has a huge development community, and countless extensions and instruments.
Python is a powerful tool when it comes to dealing with big data, data analysis, and complex calculations.
End Line
The most common Python advantages for FinTech app development with built-in AI-powered fraud detection are:
- It is faster and cheaper to find and hire Python developers, familiar with technologies and industry
- Many proven and available instruments, extensions, or consistent technologies are countless, starting with numerous Python frameworks and libraries, and ending with third-party software.
- A lot of successful niche-specific cases of using Python by financial companies, both for software products and AI development.